The NVIDIA cuSPARSELt update expands the high-performance CUDA library support for vectors of alpha and beta scalars, GeLu scaling, Split-K Mode, and more.
Month: June 2022
Firstly, I began to train the network with around 400 hundred images for 50k steps. Then, I decided to continue with the training with a new dataset with the same classes, but increased the number of steps to 110k steps; 2 more data augmentation options; dropout set to true and increased batch size from 32 to 64. It started with these loss values: loss/localization loss=1.148414 Loss/regularization loss=3695957000.0 Loss/ classification loss=508.7694 Loss/total loss=3695957500.0
Several hundred steps have passed and the losses seem to be decreasing.
Should I be worried about it starting with such high loss?
Thank you
submitted by /u/Emergency_Egg_9497
[visit reddit] [comments]
submitted by /u/Appropriate-Tap3103
[visit reddit] [comments]
If the King of Sweden wants help drafting his annual Christmas speech this year, he could ask the same AI model that’s available to his 10 million subjects. As a test, researchers prompted the model, called GPT-SW3, to draft one of the royal messages, and it did a pretty good job, according to Magnus Sahlgren, Read article >
The post The King’s Swedish: AI Rewrites the Book in Scandinavia appeared first on NVIDIA Blog.
I am trying to use Tensorflow-lite to run inference on a video frame by frame. This is my code so far:
#include <iostream> #include "src/VideoProcessing.h" #include <cstdio> #include <opencv2/opencv.hpp> #include <opencv2/videoio.hpp> #include "tensorflow/lite/interpreter.h" #include "tensorflow/lite/kernels/register.h" #include "tensorflow/lite/model_builder.h" #include "tensorflow/lite/interpreter_builder.h" int main() { int fps = VideoProcessing::getFPS("trainer.mp4"); unsigned long size = VideoProcessing::getSize("trainer.mp4"); cv::VideoCapture cap("trainer.mp4"); //Check if input video exists if(!cap.isOpened()){ std::cout<<"Error opening video stream or file"<<std::endl; return -1; } //Create a window to show input video cv::namedWindow("input video", cv::WINDOW_NORMAL); //Keep playing video until video is completed while(true){ cv::Mat frame; //Capture frame by frame cap >> frame; //If frame is empty then break the loop if(frame.empty()){break;} //Show the current frame imshow("input video", frame); } //Close window after input video is completed cap.release(); //Destroy all the opened windows cv::destroyAllWindows(); std::cout << "Video file FPS: " << fps << std::endl; std::cout << "Video file size: " << size << std::endl; // Load the model std::unique_ptr<tflite::FlatBufferModel> model = tflite::FlatBufferModel::BuildFromFile("pose_landmark_full.tflite"); // Build the interpreter tflite::ops::builtin::BuiltinOpResolver resolver; std::unique_ptr<tflite::Interpreter> interpreter; tflite::InterpreterBuilder(*model, resolver)(&interpreter); if (interpreter == nullptr) { fprintf(stderr, "Failed to initiate the interpretern"); exit(-1); } return 0; }
I use this command to run my project:
g++ -std=c++17 main.cpp src/VideoProcessing.cpp `pkg-config --libs --cflags opencv4` -o result
My tensorflow-lite is in `/usr/local/include/tensorflow/lite/`. This is my output:
In file included from /usr/local/include/tensorflow/lite/model.h:21, from /usr/local/include/tensorflow/lite/kernels/register.h:18, from main.cpp:7: /usr/local/include/tensorflow/lite/interpreter_builder.h:26:10: fatal error: flatbuffers/flatbuffers.h: No such file or directory 26 | #include "flatbuffers/flatbuffers.h" // from u/flatbuffers | ^~~~~~~~~~~~~~~~~~~~~~~~~~~ compilation terminated.
submitted by /u/janissary2016
[visit reddit] [comments]
image classification problem
Why would a trained model that performs with around 95% accuracy have worse accuracy after Transforming, duplicating & vertically flipping the original training data & adding it in with the original data and retraining it?
submitted by /u/Nothemagain
[visit reddit] [comments]
How to start learning tensorflow
Hello, so I am currently trying to learn TensorFlow and deep learning this month since we have an upcoming thesis proposal next semester and I am somewhat interested in proposing a thesis that will implement machine learning in it. To be specific, my envisioned thesis proposal will be about the early detection of pests in plants so that there will be a way for farmers to somewhat predict the conditions of their crops. I think that image recognition using machine learning can help me with this since I have read that there are a lot of things image recognition can do. Although my knowledge about machine learning and AI is very futile, especially the details in it like the Math involved and creating my own model from scratch for implementation of my envisioned topic. Tho I have heard from my colleagues, which were like me that has a proposal that involves machine learning and started doing their thesis with zero knowledge about it, that there are lots of open-source models available on the internet which can help me implement my proposal. Actually, they themselves used open-source models (which implement R-CNN and YOLO algorithms) in implementing their proposal, which I think is about microplastic detection in bodies of water using image recognition. What they’ve done is they formulated and populated their own datasets about it, the gathered dataset was used to train the model they got from the internet, tho they made some tweaking.
So my concern here is that, given my prospective proposal, should I in-depth learn about TensorFlow and create a model from scratch (which actually is good since I will learn the fundamentals about ML but it will require me a lot of effort) or just try to learn open-source models available on the internet then try to tweak something in it to best fit my intended function. I am currently taking a course in Udacity which is an Intro to Tensorflow for Deep Learning, this was the prescribed overview course of the tensorflow website, and I am somewhat unsatisfied by how things worked on that course since the course somewhat bombards me of all the codes and stuff which I really don’t know since I am new to TensorFlow (tho I know things in python). They did not give such an in-depth explanation of the codes although they give somewhat a crash course on different Deep Learning concepts such as CNNs. I am overwhelmed by that course since what I just did is to follow the tutorial, actually, I just push the play button in the google colab and wait for it to run and see if its working as intended just like what’s shown in the video tutorial. I know I am expecting a lot from that course knowing that it is an overview of the ML and TF, but I am really not satisfied with what I am getting. I want to know more about Tensorflow, especially the library itself and the codes involved, I think learning the code itself while learning the concepts involved will help me grow in this field. So are there any recommendations on which resources should I gather and learn to satisfy my learning needs in this field? Also, what better ways (I actually love getting advice from people who are better than me in this field) to learn ML and TF. I am looking forward to having a good conversation with you guys and also learning a lot about this field.
submitted by /u/MapDiscombobulated65
[visit reddit] [comments]
I’m learning tensorflow, so sorry if my question is too stupid.
I’ve just bought a RTX 3070, upgrading from my old GTX 970, both for gaming and for using with tensorflow.
If I use the two GPUs, my motherboard will “split” the speed of the PCIe3 slots from 16x to 8x. So my question is: is it worth it to keep the GTX 970 along with the RTX 3070 to send a part of the tensor calculation to the 970 using Strategy? Can the 970 give me more speed for training NNs, or will the gains be negligible? Do you guys think I should keep just the RTX 3070 on the motherboard?
My PSU is powerful enough for both (1000 watts), so the question here is just about the usefulness of the GTX 970 as a second gpu for NN training in tensorflow, since it has some CUDA cores that (I suppose) could potentially be used alongside the 3070.
What do you guys think? Have any of you tried something like that?
submitted by /u/jiaminsk2
[visit reddit] [comments]
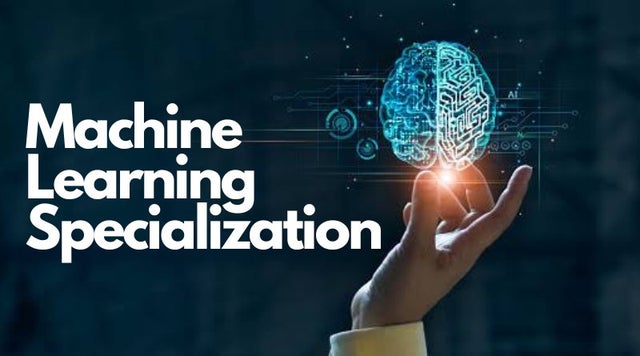
![]() |
submitted by /u/ampankajsharma [visit reddit] [comments] |
is there a default null class for training?
Is there such a thing a default null where a bunch of random real world images are used to make no choice? If we had two label classes class-1 & class-2 our model will always choose one or the other but we need a third class to say it is neither class one or class two. So there should be a default class that cross references & removes any data in this default class that correlates to either of our classes?
submitted by /u/Nothemagain
[visit reddit] [comments]