Visit NVIDIA in booth 806 at the Embedded Vision Summit 2022 and join a session to learn how the NVIDIA TAO Toolkit can help you create custom AI models without AI expertise.
Month: May 2022
Learn how you can make the most out of graphics workflows with NVIDIA RTX Desktop Manager and NVIDIA RTX Experience.
From digital content creation to product design, graphics workflows are becoming more complex, interactive, and collaborative. As many organizations around the world adjust to a hybrid work environment, designers, engineers, developers, and other professionals are constantly setting up workspaces that best suit them, no matter where they are working from.
Users can easily create optimal settings to customize their workspace and enhance productivity and efficiency with NVIDIA RTX software.
NNVIDIA RTX software has two offerings aimed at enhancing productivity:
- NVIDIA RTX Desktop Manager: users can manage single or multi-display workspaces with ease, providing maximum flexibility and control over display real estate and desktops.
- NVIDIA RTX Experience: delivers productivity tools such as driver management and content capture, to minimize context-switching over GPU tools so users can focus on their work.
Check out the top five features of NVIDIA RTX Desktop Manager and RTX Experience.
Instant, automatic downloads
Get automatic alerts from NVIDIA RTX Experience whenever a new driver is available. Instantly download and install the drivers from the application, shaving multiple steps from the normal download and install process. And if you need something from the previous driver, the rollback feature provides easy reinstallation.
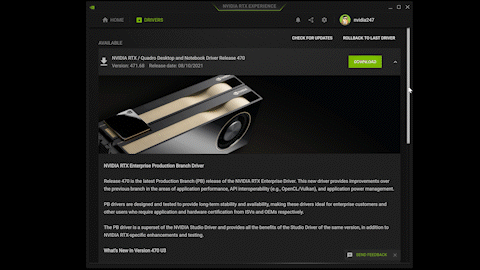
Desktop recording on demand
Use NVIDIA RTX Experience hotkeys to start recording your desktop instantly to capture images or create how-to videos to share with others. Recordings are automatically saved in a convenient repository for easy access. This is great for troubleshooting, as well.
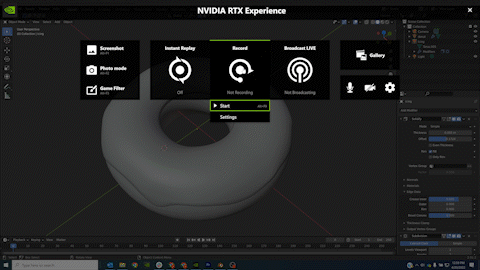
Snap Windows to grids
Use NVIDIA RTX Desktop Manager to snap windows quickly into predefined grids, and change grid configurations easily to suit specific workflows or projects. This will help maximize display real estate—while staying in tune with your aesthetics.
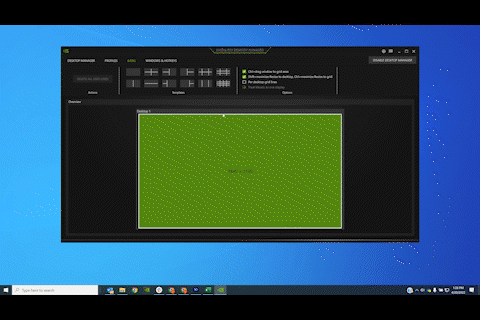
See everything from a bird’s-eye view
Manage all of your physical and virtual desktops from within the RTX Desktop Manager’s Birdseye View interface. No need to scroll or drag windows across monitors to organize and snap things into place—do it all from one central location.
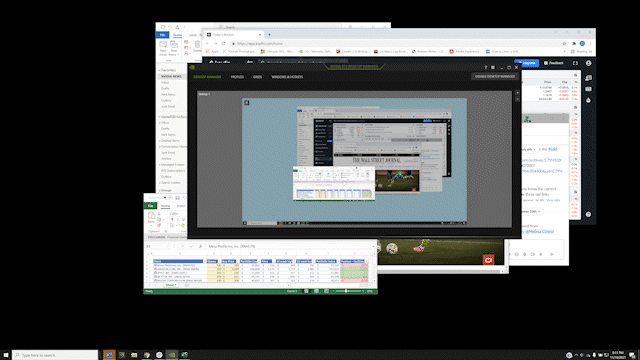
Maximize productivity with layers
Having trouble finding that app buried beneath other windows? Use the RTX Desktop Manager’s expanded toolset to toggle desired apps to ‘Always Remain on Top of the Desktop.’ You can also set the transparency level of that top window to see what is going on underneath. This is also a great trick for taking notes while on a video call.
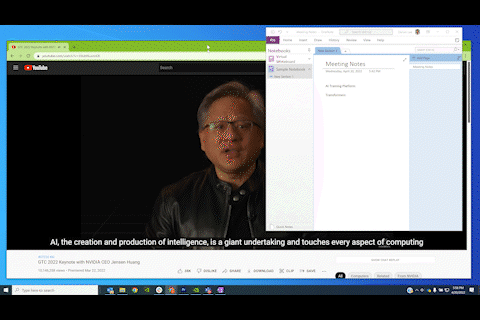
NVIDIA RTX software is available to all users who have RTX GPUs.
Download NVIDIA RTX Desktop Manager and NVIDIA RTX Experience today, and get the productivity tools to enhance work from anywhere.
NVIDIA’s latest academic collaborations in graphics research have produced a reinforcement learning model that smoothly simulates athletic moves, ultra-thin holographic glasses for virtual reality, and a real-time rendering technique for objects illuminated by hidden light sources. These projects — and over a dozen more — will be on display at SIGGRAPH 2022, taking place Aug. Read article >
The post Setting AIs on SIGGRAPH: Top Academic Researchers Collaborate With NVIDIA to Tackle Graphics’ Greatest Challenges appeared first on NVIDIA Blog.
This month the NGC catalog added a new one-click deploy feature, new speech and computer vision models, and sample speech training data to help simplify your AI app development.
The NVIDIA NGC catalog is a hub for GPU-optimized deep learning, machine learning, and HPC applications. With highly performant software containers, pretrained models, industry-specific SDKs, and Jupyter Notebooks the content helps simplify and accelerate end-to-end workflows.
New features, software, and updates to help you streamline your workflow and build your solutions faster on NGC include:
One Click Deploy
Developing AI with your favorite tool, Jupyter Notebooks, just got easier with simplified software deployment using the NGC catalog’s new one-click deploy feature.
Simply go to the software page in the NGC catalog and click on “Deploy to Vertex AI” to get started. Under the hood, this feature: launches the JupyterLab instance on Google Cloud Vertex AI Workbench with optimal configuration; preloads the software dependencies; and downloads the NGC notebook in one go. You can also change the configuration before launching the instance.
Release highlights:
- Jupyter Notebooks for popular AI use-cases.
- One Click Deploy runs NGC Jupyter Notebooks on a Google Cloud Vertex AI Workbench.
- Automated setup with optimal configuration, preloaded dependencies, and ready-to-run notebooks.
- Data scientists can focus on building production-grade models for faster time to market.
See the collection of AI software and Notebooks that you can deploy with one click.
Register for our upcoming webinar to learn how you can use our new feature to build and run your machine learning app 5X faster.
NVIDIA Virtual Machine Image
Virtual Machine Image (VMI) or AMI (in case of AWS) are like operating systems that run on top of the hypervisor on cloud platforms.
NVIDIA GPU-optimized VMI provides a standardized image across IaaS platforms so developers develop their AI application once, whether on NVIDIA-Certified Systems or any GPU cloud instance, and deploy the application on any cloud without code change.
Available from the respective cloud marketplaces, the NVIDIA VMIs are tested on NVIDIA AI software from the NGC catalog to deliver optimized performance and are updated quarterly with the latest drivers, security patches, and support for the latest GPUs.
Organizations may purchase enterprise support of NVIDIA AI software so developers can outsource technical issues and instead focus on building and running AI.
Build your AI today with NVIDIA VMI on AWS, Azure, and Google Cloud.
Deep learning software
The most popular deep learning frameworks for training and inference are updated monthly. Pull the latest version (v22.04) of:
New speech and computer vision models
We are constantly adding state-of-the-art models for a variety of speech and vision models. Here is a list of a handful of new models.
- STT Hi Conformer: Transcribes speech in Hindi characters along with spaces.
- Riva Conformer ASR Spanish: Transcribes speech in lowercase Spanish alphabet.
- EfficientNet v2-S: A family of image classification models, which achieve state-of-the-art accuracy, being an order-of magnitude smaller and faster.
- GatorTron-S: A Megatron BERT model trained on synthetic clinical discharge summaries.
- BioMegatron345m: This NeMo model delivers improved results on a range of biomedical downstream tasks.
To explore more models, visit the NGC Models page.
Sample speech training data
To help you customize pretrained models for your speech application, Defined.AI, an NVIDIA partner, is offering 30 minutes of free sample data for eight languages.
Access it now through the NGC catalog.
HPC Applications
The latest versions of popular HPC applications are also available in the NGC catalog including:
- HPC SDK: A comprehensive suite of compilers, libraries, and HPC tools.
- MATLAB: Provides algorithms, pretrained models, and apps to create, train, visualize, and optimize deep neural networks.
Visit the NGC catalog to see how the GPU-optimized software can help simplify workflows and speed up solution times.
Hello, beginner requiring help here
I’ve just picked up tensorflow and I’m trying to make a simple Siamese neural network.
How would I import csv’s as the left and right input? Any help would be greatly appreciated
def siamese_model(input_shape):
“””
Model Architecture
“””
# define the tensor for the two input texts
left_input = Input(input_shape1)
right_input = Input(input_shape2)
# convolutional neural network
model = Sequential()
model.add(Conv2D(64, (10,10),activation=’relu’,input_shape=input_shape,
kernel_initializer=initialize_weights, kernal_regularizer=12(2e-4)))
model.add(MaxPooling2D())
model.add(Conv2D(128, (7,7),activation=’relu’,
kernel_initializer=initialize_weights,
bias_initializer=initialize_bias, kernel_regularizer=12(2e-4)))
model.add(MaxPooling2D())
model.add(Conv2D(128, (4,4),activation=’relu’,
kernel_initializer=initialize_weights,
bias_initializer=initialize_bias, kernel_regularizer=12(2e-4)))
model.add(MaxPooling2D())
model.add(Conv2D(256, (4,4),activation=’relu’,
kernel_initializer=initialize_weights,
bias_initializer=initialize_bias, kernel_regularizer=12(2e-4)))
model.add(Flatten())
model.add(Dense(4096,activation=’sigmoid’,
kernel_regularizer=12(1e-3),
kernel_initializer=initialize_weights,bias_initializer=bias_initializer))
# Generate the encodings (feature vectors) for the two images
encoded_l = model(left_input)
encoded_r = model(right_input)
# Add a custom layer to compute the absolute difference between the encodings
l1_layer = Lambda(lambda tensors:K.abs(tensors[0] – tensors[1]))
l1_distance = l1_layer([encoded_l, encoded_r])
# Add a denselayer with a sigmoid unit to generate the similarity score
prediction = Dense(1,activation=’sigmoid’,bias_initializer=initialize_bias)(l1_distance)
#connect the inputs with the outputs
siamese_net = Model(inputs=[left_input, right_input],outputs=prediction)
# return model
return siamese_netamese_net
submitted by /u/Issue_647
[visit reddit] [comments]
I am trying to normalize a numpy array with tf.cast(array, tf.float32)/255.0.
When I run the script I am running into an error:
Traceback (most recent call last):
, line 91, in normalize
normalized = tf.cast(array, tf.float32) / 255.0
, line 153, in error_handler
raise e.with_traceback(filtered_tb) from None
site-packagestensorflowpythonframeworkops.py”, line 7186, in raise_from_not_ok_status
raise core._status_to_exception(e) from None # pylint: disable=protected-access
tensorflow.python.framework.errors_impl.ResourceExhaustedError: failed to allocate memory [Op:Cast]
submitted by /u/Successful-Ad-8021
[visit reddit] [comments]
How to see the CNN layer result?
Hi, I’m experimenting with CNN and I want to know if there’s a way to extract the output of a CNN layer and plot as an image to see what pattern my network identify in that layer.
submitted by /u/Current_Falcon_3187
[visit reddit] [comments]
Numpy to tfRecord
What is the best way to convert dataset from numpy to tfRecord? I try going through tensorflow documentation, it just make think worse.
submitted by /u/InternalStorm133
[visit reddit] [comments]
Over the last several years, the rapidly growing size of deep learning models has quickly exceeded the memory capacity of single accelerators. Earlier models like BERT (with a parameter size of < 1GB) can efficiently scale across accelerators by leveraging data parallelism in which model weights are duplicated across accelerators while only partitioning and distributing the training data. However, recent large models like GPT-3 (with a parameter size of 175GB) can only scale using model parallel training, where a single model is partitioned across different devices.
While model parallelism strategies make it possible to train large models, they are more complex in that they need to be specifically designed for target neural networks and compute clusters. For example, Megatron-LM uses a model parallelism strategy to split the weight matrices by rows or columns and then synchronizes results among devices. Device placement or pipeline parallelism partitions different operators in a neural network into multiple groups and the input data into micro-batches that are executed in a pipelined fashion. Model parallelism often requires significant effort from system experts to identify an optimal parallelism plan for a specific model. But doing so is too onerous for most machine learning (ML) researchers whose primary focus is to run a model and for whom the model’s performance becomes a secondary priority. As such, there remains an opportunity to automate model parallelism so that it can easily be applied to large models.
In “Alpa: Automating Inter- and Intra-Operator Parallelism for Distributed Deep Learning”, published at OSDI 2022, we describe a method for automating the complex model parallelism process. We demonstrate that with only one line of code Alpa can transform any JAX neural network into a distributed version with an optimal parallelization strategy that can be executed on a user-provided device cluster. We are also excited to release Alpa’s code to the broader research community.
Alpa Design
We begin by grouping existing ML parallelization strategies into two categories, inter-operator parallelism and intra-operator parallelism. Inter-operator parallelism assigns distinct operators to different devices (e.g., device placement) that are often accelerated with a pipeline execution schedule (e.g., pipeline parallelism). With intra-operator parallelism, which includes data parallelism (e.g., Deepspeed-Zero), operator parallelism (e.g., Megatron-LM), and expert parallelism (e.g., GShard-MoE), individual operators are split and executed on multiple devices, and often collective communication is used to synchronize the results across devices.
The difference between these two approaches maps naturally to the heterogeneity of a typical compute cluster. Inter-operator parallelism has lower communication bandwidth requirements because it is only transmitting activations between operators on different accelerators. But, it suffers from device underutilization because of its pipeline data dependency, i.e., some operators are inactive while waiting on the outputs from other operators. In contrast, intra-operator parallelism doesn’t have the data dependency issue, but requires heavier communication across devices. In a GPU cluster, the GPUs within a node have higher communication bandwidth that can accommodate intra-operator parallelism. However, GPUs across different nodes are often connected with much lower bandwidth (e.g., ethernet) so inter-operator parallelism is preferred.
By leveraging heterogeneous mapping, we design Alpa as a compiler that conducts various passes when given a computational graph and a device cluster from a user. First, the inter-operator pass slices the computational graph into subgraphs and the device cluster into submeshes (i.e., a partitioned device cluster) and identifies the best way to assign a subgraph to a submesh. Then, the intra-operator pass finds the best intra-operator parallelism plan for each pipeline stage from the inter-operator pass. Finally, the runtime orchestration pass generates a static plan that orders the computation and communication and executes the distributed computational graph on the actual device cluster.
Intra-Operator Pass
Similar to previous research (e.g., Mesh-TensorFlow and GSPMD), intra-operator parallelism partitions a tensor on a device mesh. This is shown below for a typical 3D tensor in a Transformer model with a given batch, sequence, and hidden dimensions. The batch dimension is partitioned along device mesh dimension 0 (mesh0), the hidden dimension is partitioned along mesh dimension 1 (mesh1), and the sequence dimension is replicated to each processor.
![]() |
A 3D tensor that is partitioned on a 2D device mesh. |
With the partitions of tensors in Alpa, we further define a set of parallelization strategies for each individual operator in a computational graph. We show example parallelization strategies for matrix multiplication in the figure below. Defining parallelization strategies on operators leads to possible conflicts on the partitions of tensors because one tensor can be both the output of one operator and the input of another. In this case, re-partition is needed between the two operators, which incurs additional communication costs.
![]() |
The parallelization strategies for matrix multiplication. |
Given the partitions of each operator and re-partition costs, we formulate the intra-operator pass as a Integer-Linear Programming (ILP) problem. For each operator, we define a one-hot variable vector to enumerate the partition strategies. The ILP objective is to minimize the sum of compute and communication cost (node cost) and re-partition communication cost (edge cost). The solution of the ILP translates to one specific way to partition the original computational graph.
![]() |
Inter-Operator Pass
The inter-operator pass slices the computational graph and device cluster for pipeline parallelism. As shown below, the boxes represent micro-batches of input and the pipeline stages represent a submesh executing a subgraph. The horizontal dimension represents time and shows the pipeline stage at which a micro-batch is executed. The goal of the inter-operator pass is to minimize the total execution latency, which is the sum of the entire workload execution on the device as illustrated in the figure below. Alpa uses a Dynamic Programming (DP) algorithm to minimize the total latency. The computational graph is first flattened, and then fed to the intra-operator pass where the performance of all possible partitions of the device cluster into submeshes are profiled.
![]() |
Pipeline parallelism. For a given time, this figure shows the micro-batches (colored boxes) that a partitioned device cluster and a sliced computational graph (e.g., stage 1, 2, 3) is processing. |
Runtime Orchestration
After the inter- and intra-operator parallelization strategies are complete, the runtime generates and dispatches a static sequence of execution instructions for each device submesh. These instructions include RUN a specific subgraph, SEND/RECEIVE tensors from other meshes, or DELETE a specific tensor to free the memory. The devices can execute the computational graph without other coordination by following the instructions.
Evaluation
We test Alpa with eight AWS p3.16xlarge instances, each of which has eight 16 GB V100 GPUs, for 64 total GPUs. We examine weak scaling results of growing the model size while increasing the number of GPUs. We evaluate three models: (1) the standard Transformer model (GPT); (2) the GShard-MoE model, a transformer with mixture-of-expert layers; and (3) Wide-ResNet, a significantly different model with no existing expert-designed model parallelization strategy. The performance is measured by peta-floating point operations per second (PFLOPS) achieved on the cluster.
We demonstrate that for GPT, Alpa outputs a parallelization strategy very similar to the one computed by the best existing framework, Megatron-ML, and matches its performance. For GShard-MoE, Alpa outperforms the best expert-designed baseline on GPU (i.e., Deepspeed) by up to 8x. Results for Wide-ResNet show that Alpa can generate the optimal parallelization strategy for models that have not been studied by experts. We also show the linear scaling numbers for reference.
![]() |
GPT: Alpa matches the performance of Megatron-ML, the best expert-designed framework. |
![]() |
GShard MoE: Alpa outperforms Deepspeed (the best expert-designed framework on GPU) by up to 8x. |
Conclusion
The process of designing an effective parallelization plan for distributed model-parallel deep learning has historically been a difficult and labor-intensive task. Alpa is a new framework that leverages intra- and inter-operator parallelism for automated model-parallel distributed training. We believe that Alpa will democratize distributed model-parallel learning and accelerate the development of large deep learning models. Explore the open-source code and learn more about Alpa in our paper.
Acknowledgements
Thanks to the co-authors of the paper: Lianmin Zheng, Hao Zhang, Yonghao Zhuang, Yida Wang, Danyang Zhuo, Joseph E. Gonzalez, and Ion Stoica. We would also like to thank Shibo Wang, Jinliang Wei, Yanping Huang, Yuanzhong Xu, Zhifeng Chen, Claire Cui, Naveen Kumar, Yash Katariya, Laurent El Shafey, Qiao Zhang, Yonghui Wu, Marcello Maggioni, Mingyao Yang, Michael Isard, Skye Wanderman-Milne, and David Majnemer for their collaborations to this research.
What is the best practice for splitting a dataset into train, test, and validation sets? I see all these tensorflow tutorials showing how to split data into train and test, but no mention of the validation set, which is important for hyperparameter tuning and ensuring that we can hold the test set until the end in order to get an unbiased estimate.
submitted by /u/berimbolo21
[visit reddit] [comments]